Introduction
Automated Machine Learning (AutoML) is an emerging technology that is revolutionizing the way businesses develop and deploy machine learning models. AutoML automates the entire process of building and deploying machine learning models, from data preparation to model training and deployment. This technology has the potential to reduce the time and cost associated with developing machine learning models, while also improving the accuracy and performance of the models. However, there are also challenges associated with AutoML, such as the need for a large amount of data and the potential for bias in the models. In this article, we will discuss the benefits and challenges of AutoML, and how businesses can make the most of this technology.
Overview of Automated Machine Learning: Benefits and Limitations
Automated Machine Learning (AutoML) is a rapidly emerging field of Artificial Intelligence (AI) that seeks to automate the entire process of building, deploying, and managing machine learning models. AutoML is a form of AI that uses algorithms to automate the process of creating and optimizing machine learning models. AutoML can be used to automate the process of building and optimizing machine learning models, including the selection of the best model for a given task, the selection of the best hyperparameters, and the optimization of the model’s performance.
AutoML has the potential to significantly reduce the time and effort required to build and deploy machine learning models. By automating the process of model building, AutoML can help data scientists and machine learning engineers to quickly and easily build and deploy machine learning models with minimal effort. AutoML can also help to reduce the cost of building and deploying machine learning models, as it eliminates the need for manual tuning and optimization.
AutoML also has the potential to improve the accuracy and performance of machine learning models. By automating the process of model building, AutoML can help to identify the best model for a given task, as well as the best hyperparameters for the model. This can help to improve the accuracy and performance of the model, as well as reduce the time and effort required to build and deploy the model.
However, AutoML also has some limitations. For example, AutoML is limited by the data that is available to it. AutoML can only optimize a model based on the data that is available to it, and so if the data is incomplete or of poor quality, the model may not be able to reach its full potential. Additionally, AutoML is limited by the algorithms that are used to automate the process of model building. If the algorithms are not optimized for the task at hand, the model may not be able to reach its full potential.
Overall, AutoML is a rapidly emerging field of Artificial Intelligence that has the potential to significantly reduce the time and effort required to build and deploy machine learning models. AutoML can also help to improve the accuracy and performance of machine learning models, as well as reduce the cost of building and deploying them. However, AutoML also has some limitations, such as being limited by the data that is available to it and the algorithms that are used to automate the process of model building.
Understanding the Role of Automated Machine Learning in Data Science
AutoML a variety of techniques to automate process of creating and optimizing models. These techniques include, hyperparameter optimization selection, and model. Feature engineering is the process of transforming the data to create features that can be train a machine learning model Hyperparameter optimization is the process of best set of hyperparameters for a given machine learning model. Model selection is the process of selecting the best model for given problem. Model deployment the process of deploying the to production.
AutoML can reduce the time and effort to build and deploy machine learning models. Byating the process of feature, hyperparameter optimization model selection, and model deployment, AutoML can reduce the time and effort required to build deploy machine learning models by to 90%. This can significantly reduce the time to market for new products and services.
AutoML can also reduce the risk associated building and deploying machine learning models. By automating the process of feature, hyperparameter optimization, model, and model deployment, AutoML can reduce the risk errors and mistakes that can to costly mistakes. This significantly reduce risk associated with building and machine learning models.
AutoML is an invaluable tool for data scientists and significantly reduce the time and required to build and deploy machine learning models. By automating the of feature engineering, hyperparameter optimization, model selection and model deployment, AutoML can the time and effort required build and deploy machine learning models by up to 90 This can significantly reduce the to market for new products services and reduce the risk associated with building and deploying learning models.
Advantages of Automated Machine Learning for Businesses
Automated Machine Learning (AutoML) is revolutionizing the way businesses use machine learning. AutoML is a type of artificial intelligence (AI) that automates the process of building, training, and deploying machine learning models. By automating the process, businesses can reduce the time and resources needed to develop and deploy machine learning models, resulting in faster time to market and improved performance.
AutoML can be used to develop models for a variety of tasks, including image recognition, natural language processing, and predictive analytics. By automating the process, businesses can quickly develop models that are tailored to their specific needs. AutoML also eliminates the need for manual coding, which can be time-consuming and error-prone.
AutoML can also help businesses save money. By automating the process, businesses can reduce the need for costly data scientists and other personnel. Additionally, AutoML can reduce the amount of time needed to develop and deploy models, resulting in cost savings.
AutoML can also help businesses improve the accuracy of their models. By automating the process, businesses can quickly develop models that are tailored to their specific needs. AutoML can also reduce the amount of time needed to develop and deploy models, resulting in improved accuracy.
Finally, AutoML can help businesses reduce the risk of errors. By automating the process, businesses can reduce the need for manual coding, which can be time-consuming and error-prone. Additionally, AutoML can reduce the amount of time needed to develop and deploy models, resulting in fewer errors.
Overall, AutoML is revolutionizing the way businesses use machine learning. By automating the process, businesses can reduce the time and resources needed to develop and deploy machine learning models, resulting in faster time to market and improved performance. Additionally, AutoML can help businesses save money, improve the accuracy of their models, and reduce the risk of errors.
Challenges of Automated Machine Learning Implementation
Automated Machine Learning (AutoML) is an emerging technology that promises to revolutionize the way data scientists and machine learning engineers develop models. AutoML is an automated process for building machine learning models that can be used to solve a variety of tasks. The goal of AutoML is to reduce the amount of time and effort required to build a machine learning model.
However, implementing AutoML is not without its challenges. In order to successfully implement AutoML, data scientists and machine learning engineers must be able to identify the right data, select the appropriate algorithms, and optimize the model for the best performance. Additionally, AutoML requires a significant amount of computing power, which can be expensive and difficult to obtain.
One of the biggest challenges of AutoML is data preparation. Data must be cleaned, formatted, and organized in order for the AutoML algorithms to work properly. This can be a time-consuming and labor-intensive process, especially if the data is unstructured or from multiple sources. Additionally, the data must be of high quality in order for the AutoML algorithms to work properly.
Another challenge of AutoML is algorithm selection. Data scientists and machine learning engineers must be able to identify the right algorithms for the task at hand. This can be difficult, as there are a wide variety of algorithms available and it can be difficult to determine which ones will work best for a given task. Additionally, the algorithms must be optimized for the best performance, which can be a time-consuming process.
Finally, AutoML requires a significant amount of computing power. This can be expensive and difficult to obtain, especially for smaller organizations. Additionally, AutoML algorithms can be computationally intensive, which can lead to long run times and increased costs.
Overall, implementing AutoML can be a challenging process. Data scientists and machine learning engineers must be able to identify the right data, select the appropriate algorithms, and optimize the model for the best performance. Additionally, AutoML requires a significant amount of computing power, which can be expensive and difficult to obtain. Despite these challenges, AutoML has the potential to revolutionize the way data scientists and machine learning engineers develop models.
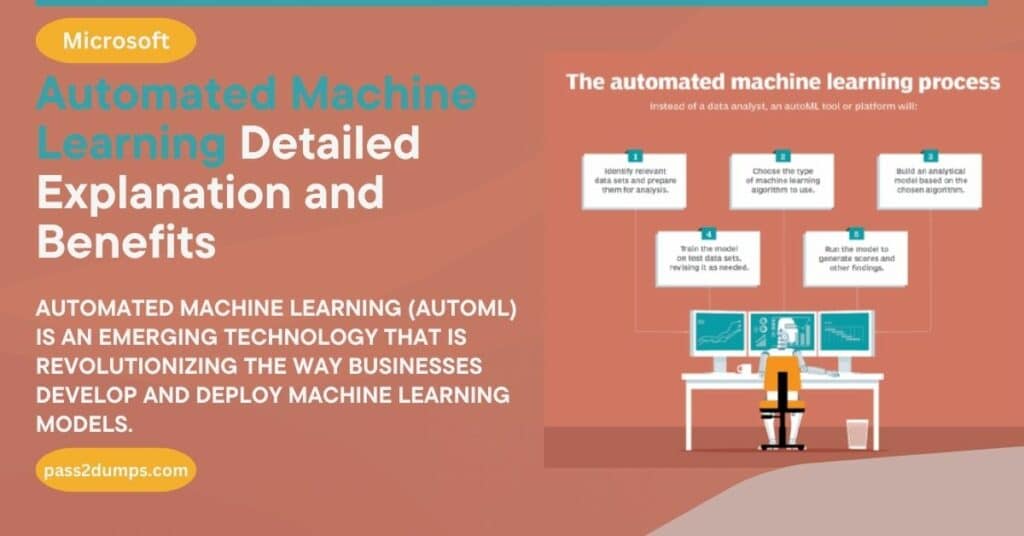
The Future of Automated Machine Learning in Business Applications
The Future of Automated Machine Learning in Business Applications is an exciting and rapidly evolving field. Automated Machine Learning (AutoML) is a technology that automates the process of creating, training, and deploying machine learning models. It enables businesses to quickly and efficiently develop and deploy machine learning models without the need for manual coding or extensive data science expertise.
AutoML is already being used in a variety of business applications, such as predictive analytics, customer segmentation, and fraud detection. It is also being used to automate the process of feature engineering, which is the process of extracting useful information from raw data. By automating this process, businesses can quickly and easily develop models that are more accurate and reliable than those developed manually.
The Future of Automated Machine Learning in Business Applications is bright. As businesses continue to adopt AutoML, the technology will become more sophisticated and powerful. This will enable businesses to develop more complex models that can better handle large datasets and more accurately predict outcomes.
AutoML is also becoming increasingly accessible. There are now a variety of AutoML platforms available, such as Google’s AutoML Vision and Microsoft’s Azure Machine Learning Studio, that make it easier for businesses to get started with AutoML. These platforms provide an intuitive interface that allows businesses to quickly and easily develop and deploy machine learning models.
The Future of Automated Machine Learning in Business Applications is also being shaped by the emergence of new technologies, such as deep learning and reinforcement learning. Deep learning is a type of machine learning that uses artificial neural networks to identify patterns in data. Reinforcement learning is a type of machine learning that uses rewards and punishments to learn how to take the best action in a given situation. Both of these technologies are being used to develop more powerful and accurate machine learning models.
The Future of Automated Machine Learning in Business Applications is an exciting and rapidly evolving field. As businesses continue to adopt AutoML, the technology will become more powerful and accessible, enabling businesses to develop more accurate and reliable models. This will enable businesses to make better decisions, improve customer experiences, and gain a competitive edge.
Conclusion
Automated Machine Learning (AutoML) offers many potential benefits, including improved accuracy, faster model development, and increased scalability. However, there are also challenges associated with AutoML, such as the need for data preparation, the complexity of feature engineering, and the potential for overfitting. By understanding the benefits and challenges of AutoML, organizations can make informed decisions about whether to use AutoML in their data science projects.
What is Microsoft 365? and How it is Helpful for You?
[…] 365 is a comprehensive suite of cloud-based services and applications designed to help individuals and businesses of all sizes work smarter and more efficiently. It combines the power of Office 365, Windows 10, […]