Key Takeaways:
- Data analysis is indispensable for informed decision-making in any sector.
- Understanding the data analysis process, techniques, and tools is essential for drawing meaningful insights.
- Exploring, visualizing, and interpreting data are powerful steps toward uncovering patterns and trends.
- Statistical methods and machine learning offer robust approaches to analyzing and predicting outcomes.
- Effectively communicating data analysis results is crucial for informing decisions and driving action.
Question: How can businesses leverage data analysis to make informed decisions?
Answer: Businesses can harness data analysis to make informed decisions by understanding the data analysis process, utilizing techniques like exploring, visualizing, and interpreting data, employing statistical methods and machine learning for analysis and prediction, and effectively communicating results to drive action and optimize decision-making processes.
Introduction
Data analysis is an essential skill for any business or organization to have. It allows you to make informed decisions based on data-driven insights. This comprehensive guide to data analysis provides an introduction to the fundamentals of data analysis, including an overview of the data analysis process, the types of data analysis, and the tools and techniques used in data analysis. It also covers topics such as data visualization, data mining, and machine learning. With this guide, you will gain a better understanding of the data analysis process and how to use it to your advantage.
Overview of Data Analysis Techniques
Data analysis is the process of collecting, organizing, and interpreting data to gain insights and draw conclusions. It is an essential component of any research project and is used to identify patterns, trends, and relationships in data.
Data analysis techniques can be divided into two main categories: quantitative and qualitative. Quantitative techniques involve the use of numerical data to conclude, while qualitative techniques involve the use of descriptive data to conclude.
Quantitative data analysis techniques include descriptive statistics, inferential statistics, and predictive analytics. Descriptive statistics are used to summarize and describe data. This includes measures of central tendency such as the mean, median, and mode, as well as measures of dispersion such as the range, variance, and standard deviation. Inferential statistics are used to make inferences about a population based on a sample. This includes tests of significance such as the t-test and chi-square test. Predictive analytics is used to develop models that can predict future outcomes based on past data.
Qualitative data analysis techniques include content analysis, thematic analysis, and discourse analysis. Content analysis is used to analyze the content of text or other media. This includes identifying themes, patterns, and relationships in the data. Thematic analysis is used to identify and analyze the underlying themes in a dataset. Discourse analysis is used to analyze the way people communicate and the language they use.
Data analysis techniques are used to draw meaningful insights from data. They are used in a variety of fields, including business, economics, sociology, psychology, and medicine. They are also used in data science, where they are used to develop models and algorithms that can be used to make predictions and decisions.
Exploring and Visualizing Data
Exploring and visualizing data is a powerful way to gain insights into complex data sets. It is a process of analyzing and interpreting data to uncover patterns, trends, and relationships that may not be immediately apparent. Through this process, data can be presented in a more meaningful way, allowing for more informed decisions and a better understanding of the data.
Exploring and visualizing data involves a variety of techniques and tools. Data exploration is the process of analyzing and understanding the data, often through visualizations such as charts, graphs, and maps. This helps to identify patterns, trends, and relationships in the data. Data visualization is the process of creating visual representations of data, such as charts, graphs, and maps, to make the data easier to understand and interpret.
Exploring and visualizing data can be used to gain insights into a variety of topics, from business performance to consumer behavior. It can be used to identify correlations between different variables, uncover hidden patterns, and gain a better understanding of the data. It can also be used to identify trends and outliers in the data, as well as to make predictions about future outcomes.
Exploring and visualizing data can be a powerful tool for making informed decisions. By analyzing and interpreting data, organizations can gain insights into their data that can be used to inform decisions and strategies. It can also be used to identify areas of improvement and opportunities for growth.
Exploring and visualizing data is an important part of data analysis and is a valuable tool for gaining insights into complex data sets. Through the use of data exploration and visualization techniques, organizations can gain a better understanding of their data and make more informed decisions.
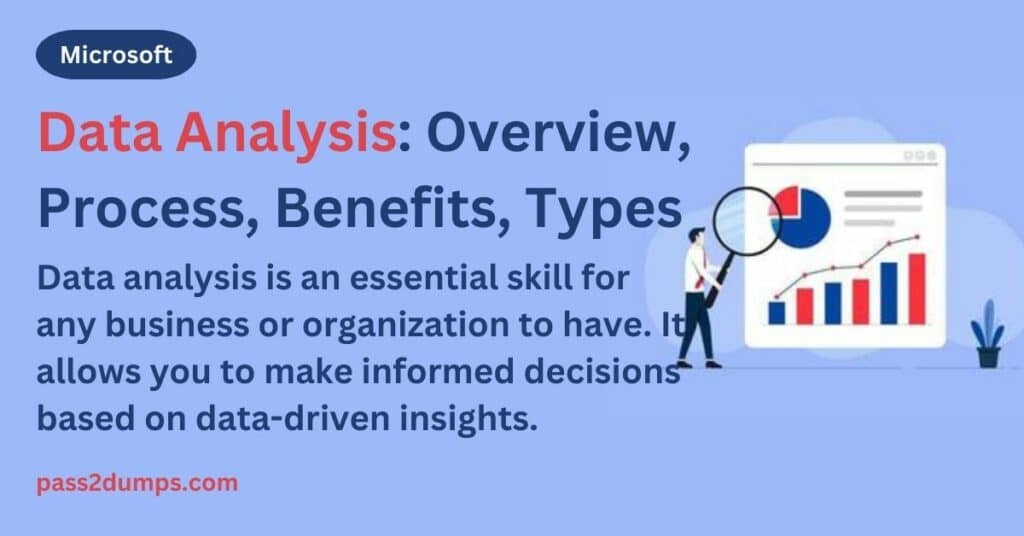
Analyzing Data with Statistical Methods
Analyzing data with statistical methods is a powerful tool for understanding the underlying patterns and relationships within a given dataset. Statistical methods allow us to make meaningful conclusions about the data by identifying patterns, trends, and correlations. Statistical methods can be used to make predictions, assess risk, and optimize decision-making.
The first step in analyzing data with statistical methods is to collect the data. This data can come from a variety of sources, such as surveys, experiments, or observations. Once the data is collected, it is important to organize it into a format that can be easily analyzed. This may involve sorting the data into categories, creating a spreadsheet, or using a statistical software package.
Once the data is organized, it is important to identify the type of statistical analysis that is most appropriate for the data. This will depend on the type of data and the research question being asked. Common types of statistical analysis include descriptive statistics, inferential statistics, and predictive analytics.
Descriptive statistics are used to summarize the data and describe the characteristics of the data. This includes measures of central tendency (mean, median, and mode) and measures of variability (standard deviation and range). Descriptive statistics can be used to identify patterns and trends in the data.
Inferential statistics are used to make predictions and draw conclusions about the data. This includes hypothesis testing, correlation, and regression analysis. Inferential statistics can be used to assess the strength of relationships between variables and make predictions about future outcomes.
Predictive analytics are used to identify patterns in the data and make predictions about future outcomes. This includes machine learning algorithms, such as decision trees, random forests, and neural networks. Predictive analytics can be used to optimize decision-making and identify opportunities for improvement.
Analyzing data with statistical methods is a powerful tool for understanding the underlying patterns and relationships within a given dataset. By using the appropriate statistical methods, researchers can make meaningful conclusions about the data and optimize decision-making.
Machine Learning for Data Analysis
Machine Learning for Data Analysis is a powerful tool that can be used to uncover hidden patterns and insights from large datasets. It is a type of artificial intelligence (AI) that uses algorithms to analyze data and make predictions or decisions based on the results.
Machine Learning for Data Analysis is used in a variety of industries, from healthcare to finance, to help organizations make better decisions. It can be used to detect fraud, identify customer trends, and even predict future events. It is also used to improve customer service, reduce costs, and increase efficiency.
The process of Machine Learning for Data Analysis begins with collecting and organizing data. This data is then used to create a model, which is a mathematical representation of the data. The model is then used to make predictions or decisions based on the data.
The model is then tested to ensure it is accurate and reliable. This is done by running the model on a test dataset and comparing the results to the actual data. If the model is accurate, it can be used to make predictions or decisions on new data.
The process of Machine Learning for Data Analysis is highly complex and requires a great deal of expertise. It is important to have an experienced data scientist or analyst on hand to ensure the accuracy of the model.
Machine Learning for Data Analysis is a powerful tool that can be used to uncover hidden patterns and insights from large datasets. It is used in a variety of industries to help organizations make better decisions and improve customer service, reduce costs, and increase efficiency. With the right expertise, Machine Learning for Data Analysis can be a powerful tool for any organization.
Interpreting and Communicating Results of Data Analysis
Interpreting and communicating the results of data analysis is a critical part of any data analysis project. This process involves taking the data collected, analyzing it, and then presenting the results in a way that is meaningful and understandable to the audience.
The first step in interpreting and communicating the results of data analysis is to identify the key findings. This involves looking at the data and determining which results are most important and relevant to the project. Once the key findings have been identified, the analyst must then interpret the data and explain what it means. This involves looking at the data from different angles and considering how the results might be used to inform decisions or to gain insights into the project.
Once the data has been interpreted, the analyst must then communicate the results. This involves presenting the data in a way that is understandable to the audience. This could involve creating charts, graphs, or other visual representations of the data. It could also involve writing a report or presenting the results in a meeting.
The goal of interpreting and communicating the results of data analysis is to ensure that the data is understood and used effectively. This process requires an understanding of the data, an ability to interpret it, and an ability to communicate the results in a way that is meaningful and understandable to the audience. It is an important part of any data analysis project and should not be overlooked.
Conclusion
In conclusion, Introduction to Data Analysis: A Comprehensive Guide is an invaluable resource for anyone looking to gain a better understanding of data analysis. It provides a comprehensive overview of the fundamentals of data analysis, including data collection, cleaning, analysis, and visualization. With its easy-to-follow instructions and detailed explanations, this guide is an excellent resource for beginners and experienced data analysts alike. With its comprehensive coverage of the fundamentals of data analysis, this guide is an essential tool for anyone looking to become an expert in the field.
BCBA Dumps Certified In BACB Braindumps With Our Dumps 2024
[…] Free Three-Month Regular Update On BCBA Dumps […]